How Is the Healthcare Sector Being Revolutionized by Machine Learning?
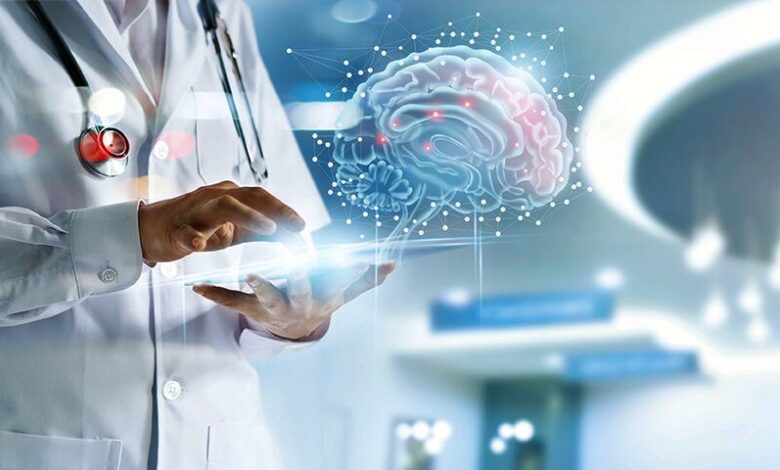
How Is the Healthcare Sector Being Revolutionized by Machine Learning?
Machine learning is set to change the healthcare sector. What if you were informed that machines would soon carry out surgery? Yes, machine learning has improved quickly to the point that it may soon be possible to execute medical procedures with little to no assistance from a doctor. By 2022, machine learning will be employed extensively in the healthcare sector.
The first thing that springs to mind when one hears the words artificial intelligence or machine learning is robots, but machine learning is far more involved than that. Machine learning has advanced in every conceivable industry and transformed numerous businesses, including finance, retail, and healthcare. This article will discuss how machine learning changes the healthcare sector. So let’s get down to business right away.
What Is Machine Learning?
With machine learning and artificial intelligence application, a system can learn from its mistakes and improve over time. The main aim is to enable computers to learn autonomously, without any help from human input. Data observations, pattern discovery, and future decision-making are the first steps in the learning process. In India, machine learning has begun to take hold.
How does Machine Learning work?
A fundamental component of artificial intelligence is machine learning, which enables computers to learn from the past and predict the future.
Data exploration and pattern matching are involved, with little assistance from humans. Machine learning has mainly been used in four technologies:
1. Supervised Learning:
A machine learning technique called “supervised learning” necessitates monitoring as a student-teacher interaction does. In supervised learning, a machine is trained using data that has already been correctly labelled with some of the outputs. As a result, supervised learning algorithms assess sample data whenever new data is entered into the system and use that labelled data to predict accurate results.
It is classified into two different categories of algorithms:
- Classification: It deals with when output is in the form of a category such as Yellow, blue, right or wrong, etc.
- Regression: It deals with when output variables are real values like age, height, etc.
Because of technology, individuals are able to collect or produce data based on experience. Utilising certain labelled data points from the training set, it operates similarly to how people learn. It assists in addressing various challenging computational issues and optimising the performance of models utilising experience.
2. Unsupervised Learning:
Unlike supervised learning, unsupervised learning allows a machine to be trained without needing to classify or clearly label data. Even without any labelled training data, it seeks to create groups of unsorted data based on some patterns and differences. Since there is no supervision in unsupervised learning, the machines are not given any sample data. As a result, robots can only discover hidden structures in unlabeled data.
It is classified into two different categories of algorithms:
- Clustering: In training data, there is a need for inherent groupings, such as categorising students according to their areas of interest.
- Association: It deals with the principles that aid in identifying a sizable chunk of data, such as students interested in both ML and AI.
3. Semi-supervised learning:
Combining supervised and unsupervised learning techniques is known as semi-supervised learning. It is used to get around both supervised and unsupervised learning’s shortcomings.
In the semi-supervised learning approach, both labelled and unlabelled data are used to train the machine. Nevertheless, it includes a sizable number of unlabeled cases and a few examples with labels. Some of the most well-known semi-supervised learning real-world applications are speech analysis, web content classification, protein sequence classification, and text document classifiers.
4. Reinforcement Learning:
A feedback-based machine learning technique known as reinforcement learning excludes the need for labelled data. An agent learns how to behave, thereby performing actions and observing how they affect the environment. Agents can offer compliments for each constructive action and criticism for destructive ones. Since there are no training data for reinforcement learning, agents can only learn from their experience.
Importance of Machine Learning
Even though constantly new technologies are emerging, machine learning is still utilised in several different industries.
Machine learning is essential because it helps companies create new products and gives them a picture of consumer behaviour trends and operational business patterns.
Machine learning is fundamental to the operations of many of the leading businesses of today, like Facebook, Google, and Uber. Machine learning has become a major point of competitive difference for many firms.
Machine learning has a number of real-world uses that produce tangible business outcomes, including time and money savings, that could significantly impact your company’s future. One mainly observes a significant impact on the customer care sector, where machine learning enables humans to complete tasks more quickly and effectively. Through Virtual Assistant solutions, machine learning automates actions that would usually require a human person to complete them, including resetting a password or checking an account’s balance. By doing this, valuable agent time is freed up so they can concentrate on the high-touch, complex decision-making tasks that humans excel at but that machines struggle with.
Machine Learning in Healthcare
There have been many breakthroughs in the healthcare sector, but machine learning has improved the efficiency of healthcare firms.
Although machine learning has come a long way, a doctor’s brain remains the best machine learning tool in the healthcare sector. Many doctors are concerned that machine learning will take over the healthcare sector.
The focus should be placed on how doctors may utilise machine learning as a tool to enhance clinical use and supplement patient care. Even if machine learning completely replaces doctors, patients would still require a human touch and attentive care.
How is Machine Learning Used in Healthcare?
Machine learning is making inroads into several businesses, and this trend appears to continue indefinitely. Additionally, it has begun to demonstrate its abilities in the healthcare sector. Some of the ways it is used there are:
-
Identifying Disease and Diagnosis
Machine learning algorithms that forecast disease or enable early disease and illness diagnoses are already under development by scientists. Artificial intelligence algorithms are being developed by the technological startup feebris, based in the UK, to identify complicated respiratory disorders accurately. The Computer Science and Artificial Intelligence Lab at MIT have created a novel deep learning-based prediction model that can forecast the onset of breast cancer up to five years in the future.
-
Robotic Surgery
Since it started in 1980, the use of robotics in healthcare has been expanding quickly. Although many people still find the idea of a robot performing surgery unsettling, it will soon become a normal practice.
In hospitals, robotics is also utilised to monitor the patients and notify the nurses when human interaction is necessary.
The robotic assistant can find the blood vessel and take the patient’s blood with minimal discomfort and concern. In pharmaceutical labs, robots also dispense and prepare drugs and vaccinations. Robotic carts are utilised in large facilities to transport medical supplies. Speaking about humans being replaced by robots, that won’t be happening anytime soon; robotics can only help doctors, never taking their place.
Medical Imaging Diagnosis
The procedure or technique known as medical imaging diagnostic involves creating a visual depiction of tissue or internal organ parts to monitor health, diagnose, and treat disorders. Additionally, it aids in the creation of an anatomy and physiology database. Using medical imaging technology like ultrasound and MRI can prevent the need for surgical procedures.
Uses of Machine Learning in the Healthcare Industry
Machine learning algorithms are properly taught to recognise the subtleties in CT scans and MRIs and can handle enormous amounts of medical pictures quickly. A deep learning team has developed an algorithm from the US, France, and Germany that can diagnose skin cancer more precisely than a dermatologist.
Pros of Machine Learning in Healthcare
Because of its advantages, machine learning is becoming increasingly popular among healthcare organisations. Several advantages include:
-
Patterns Are Easily Identified
The ability of machine learning to precisely recognise patterns and data, which may be impossible for a human to do, is one of its greatest strengths. It can quickly and efficiently process enormous amounts of data and patterns. All of these are feasible with the new invention.
-
Smart Health Records
Because maintaining health data requires a lot of work, machine learning is used to streamline the procedure and reduce the time and effort needed. Machine learning is developing cutting-edge technology for keeping smart data records in the modern world.
-
Minimum Human Intervention
By gaining knowledge from patterns and data over time, machine learning adapts. The main advantage of machine learning is that it can easily execute procedures and requires little human interaction.
Cons of Machine Learning in Healthcare
There is also some uncertainty because, despite its advantages, machine learning also has drawbacks. Among them are:
-
Data Acquisition
Machine learning trains its algorithms using enormous data sets and patterns since it adapts through patterns and data settings. The information must be accurate and of high calibre.
-
Take Time to Learn and Adapt
For machine learning to produce correct results, it needs enough time for its algorithms to absorb and adjust to the patterns and data. It functions better with more computing power.
-
High Error-Susceptibility
Machine learning is extremely error-prone, necessitates a vast quantity of data, and may not perform as intended if not given enough of it. Any inaccurate data fed to the machine may result in an undesirable result.
Future of Machine Learning in Healthcare
The advancement of machine learning will enable the automatic early detection of most ailments. It will also improve the efficiency and accuracy of disease detection to lessen the strain on doctors. Future healthcare will change thanks to AI and machine learning.
Machine learning has grown quickly in every industry, including navigation, business, retail, and banking. However, success in the healthcare sector is challenging due to the scarcity of high-calibre scientists and the limited availability of data. Numerous elements, including machine learning, still need to be addressed.
Conclusion
The use of machine learning in the healthcare sector has increased in popularity and usage. By simplifying their tasks, ML benefits patients and physicians in various ways. Automating medical billing, offering clinical decision support, and creating clinical care standards are some of the most popular uses of machine learning. Machine learning has numerous applications that are currently being investigated and developed.
Future developments in machine learning (ML) applications in the healthcare industry will greatly improve the quality of life for people.
Edited by Prakriti Arora